According to WHO, depression is becoming a leading cause of disability. The aim of the “Reducing the rate and duration of readmission among patients with unipolar and bipolar disorder using smartphone-based monitoring and treatment” (RADMIS) project is to design smartphone-based monitoring and treatment technology for depressive patients. The clinical goal is to establish the efficacy of this technology by measuring hospital re-admission and clinical outcome.
Background
Depression and bipolar disorder (affective disorders) impose a very high societal burden in terms of cost, lost productivity, morbidity, suffering, and mortality, and is according to WHO a leading cause of disability and disease burden worldwide. According to the European Commission, depression is among the most pressing public health concern today, and account for more than 12% of all estimated ill health and premature mortality in Europe, only exceeded by heart disease and cancer.
Although psychiatric treatment internationally and in Denmark has shifted more from inpatient treatment to outpatient treatment during recent decades, costs to psychiatric hospitalization are still significant. In Denmark, two third of all direct costs within psychiatry is related to hospitalization. Patients with affective disorders are more frequently hospitalized than any other patient group, counting more than 10.800 patients in 2013 and 20% of all psychiatric hospitalizations.
Objectives
Smartphones provide a unique platform for monitoring and treatment of depression and mania (see e.g this review of a Decade of Ubicomp Research in Mental Health [1]) . Equipped with powerful sensing, computation and communication capabilities, smartphones can continuously monitor an individual’s context including physical activity, location and environment. Depression and mania are associated with several behavioral components (e.g., reduction in activity, change in speech, changes in sleep) and motivational states (e.g., anhedonia), some of which may be detectable using smartphone sensors. Thus, smartphones hold significant promise as a platform to monitor behavioral and environmental indicators of depression and mania (see also the MONARCA project).
Moreover, a smartphone also provides a rich multi-media and communication platform for Ecological Momentary Intervention (EMI), i.e. the delivery of context-aware and real-time treatment, including medication reminders and cognitive behavioral therapy (CBT). This intervention utilize the data monitored. The objective of the RADMIS project is to design, implement, and evaluate a platform for delivery of such just-in-time, context-aware treatment for patients with depression.
In summary, the RADMIS project have three overall research strands, which run concurrently and intertwined:
- Research, design, and develop smartphone-based CBT monitoring and treatment technology for depressive disorders.
- Establish methods and algorithms for efficient and scalable data processing and analysis of behavioral data aiming at pattern recognition and disease prediction of recurring depressive and manic phases.
- To establish clinical evidence for (a) reduction of re-hospitalization and (b) improving depression and other outcomes for patients with unipolar and bipolar depression receiving smartphone-based CBT.
Results
The results from the RADMIS projet have been reported in a number of peer-reviewed scientific papers, which are all included in the reference list at the end of this post. The following sections provide an overview of these results, while the reader is referred to the papers for details.
#1 – Smartphone-based Monitoring and Treatment Technology
The first part of the RADMIS project was focusing on the design of smartphone-based technology for cognitive behavioral therapy (CBT). In this research, we focused on the behavioral part of CBT, i.e. helping depressive patients to structure and adopt healthy behavior. The design took its outset in Behavioral Activation (BA), which is a 3rd generation behavior therapy for treating depression.
In a systematic literature review [2] we analysed the correlation between objective behavioral features collected via mobile and wearable devices and depressive mood symptoms in patients.
This review provides an overview of the correlations between objective behavioral features and depressive mood symptoms as reported in the literature, and investigates the strength and statistical significance of these correlations across studies.
A total of 46 studies, 85 unique objective behavioral features, and 17 various sensor data inputs were included in the review. Several studies showed consistent and statistically significant correlations between objective behavioral features collected via mobile and wearable devices and depressive mood symptoms. Figure 1 shows which feature have a strong correlation, and shows, for example, that phone calls and mobility (as tracked by cell tower ids) have a strong negative correlation with depressive symptoms, whereas screen activity and sleep duration have a strong positive correlation.
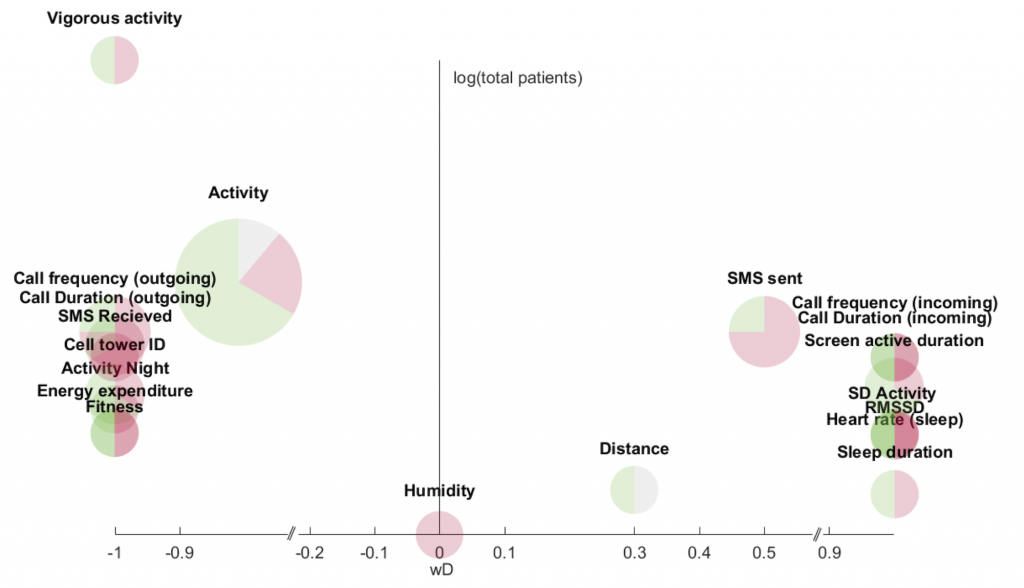
The review concludes that continuous and everyday monitoring of behavior is a promising objective measure for estimating depressive mood symptoms.
In order to understand the impact of behavior on the mood of patients, a detailed analysis of behavioral activation records were done [3, 4]. The goal was to (a) automatically identify behavioral patterns through statistical analysis of the paper-based activity diaries, and (b) determine whether it is feasible to move the behavioral activation therapy format to a digital solution. We collected activity diaries from seven patients with bipolar depression, covering in total 2,480 hours of self-reported activities.
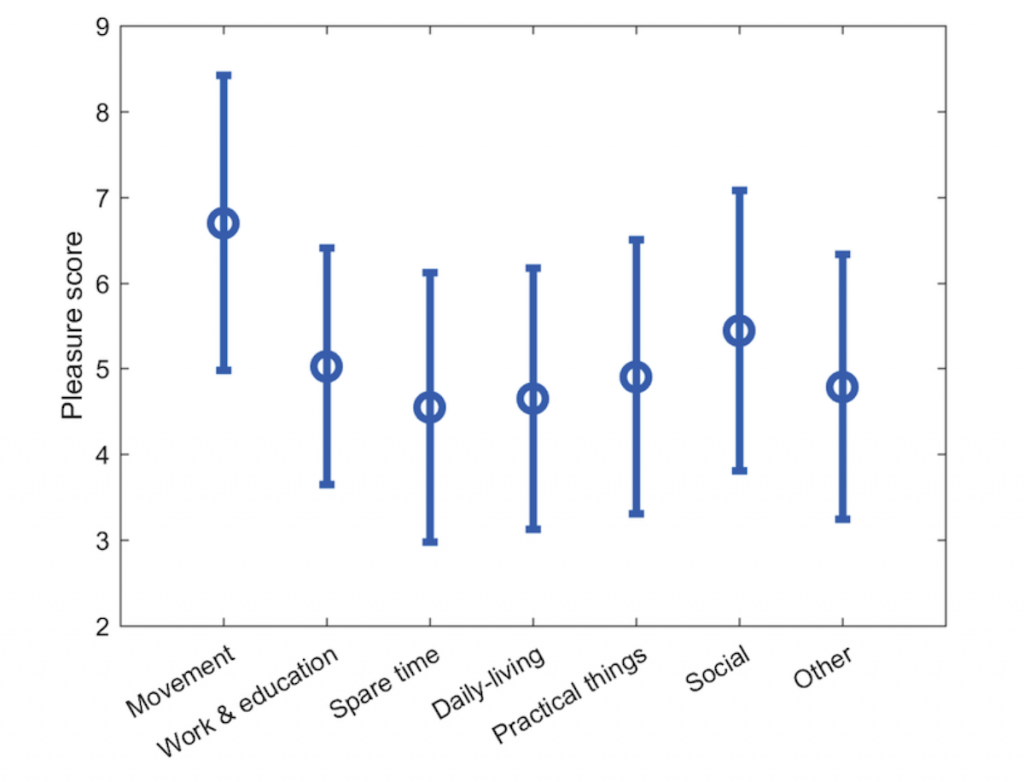
As shown in Figure 2, across all patients, movement-related activities were associated with the highest pleasure score followed by social activities. Furthermore, the patients were found to have a better day than the previous, if that previous day followed their circadian rhythm and a second-order analysis indicated that two hours of daily social activity was optimal for the patients.
Based on these analyses, a user-centred design process was initiated to design Behavioral Activation Technologies. This resultet in two smartphone-based systems:
- MORIBUS [5] – This system is designed for detailed hourly activity planning and registration, which is a core ingredient in BA therapy. Normally, patients are asked to do this in a paper-based diary (see e.g. [3, 4]) and MORIBUS is an app replacing – and enhancing — this approach. MORIBUS is designed to be part of BA therapy (and not replace it), and is hence designed to be used in close collaboration with a therapist.
- MUBS [6] – This system is designed for more coarse-grained activity planning and registration. It applies advanced AI technology for recommendation of activities based on a personalized profile. MUBS is designed to be used by patients ‘stand-alone’, i.e. not necessarily as part of BA therapy.
These technologies for behavioral activation is further described in a separate blog post.
Both systems were subject to a clinical feasibility study [7] where the systems’ feasibility was investigated in terms of usage adoption, usability, usefulness, and clinical outcomes. The study of MORIBUS [5] included seven patients who planned in total 1,684 activities across 140 days. Overall study compliance was 71% as calculated by the number of days that activities were registered or rated within the entire study duration. In the study, patients reported that they found MORIBUS easy to use and very useful for supporting BA therapy. Most patient reported that they preferred MORIBUS (i.e., a smartphone app) over the paper-based diaries they normally used. Moreover, the study revealed quite individual activity patterns, as shown in Figure 3 which shows the overall distribution of activities within the seven activity categories – a lesson that is important to incorporate into the design of such BA technology.
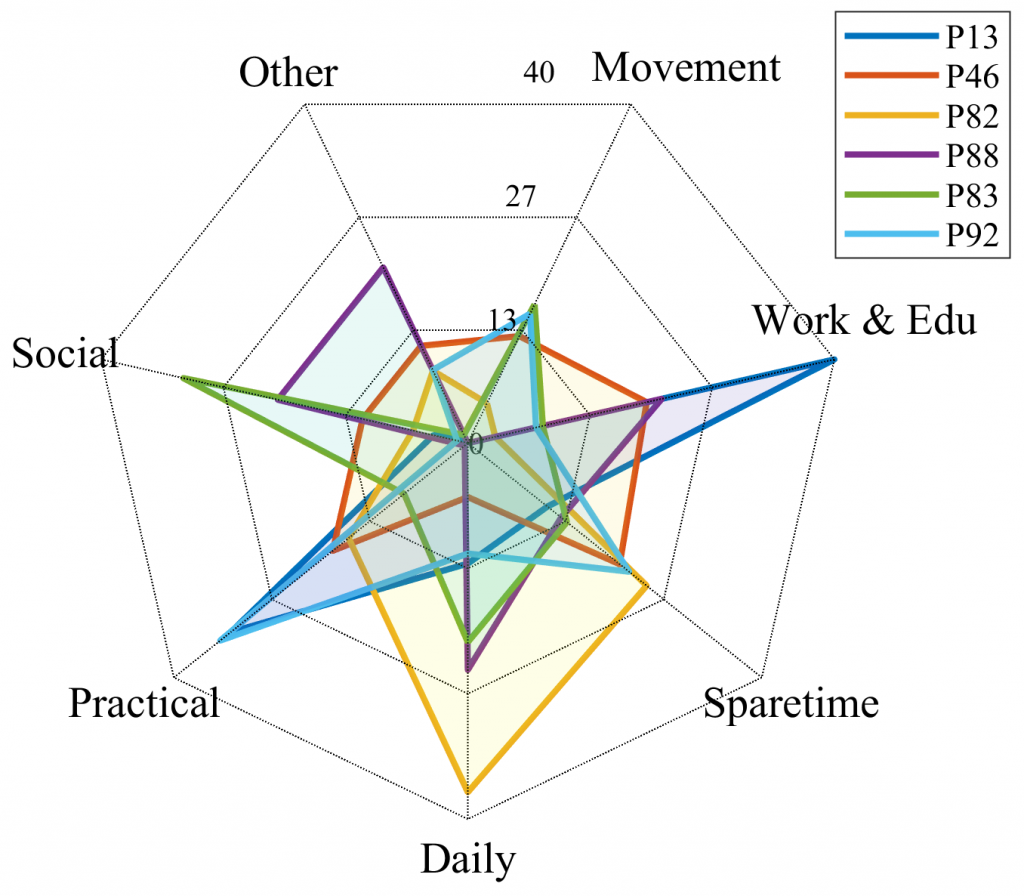
An 8-week feasibility study of MUBS [6] involving 17 depressive patients provided detailed insight into how MUBS provided inspiration and motivation for planning and engaging in more pleasant activities, thereby facilitating the core components of BA. Overall, study compliance was 76% as calculated by the number of days that MUBS were used for activity planning within the entire study duration. The study showed that a majority of patients believed that using MUBS would help attain gains in health. For example, 75% of patients agreed to the statement that “using MUBS would help me reach my health goals of reducing depressive symptoms”.
A subsequent 8-week clinical feasibility study involving 29 patients diagnosed with a depressive disorder reports on clinical outcome and usage patterns [8]. In this study, we observed a statistically significant reduction in depressive symptom severity during the study as shown in figure 4 below (as measured using the PHQ questionnaire).
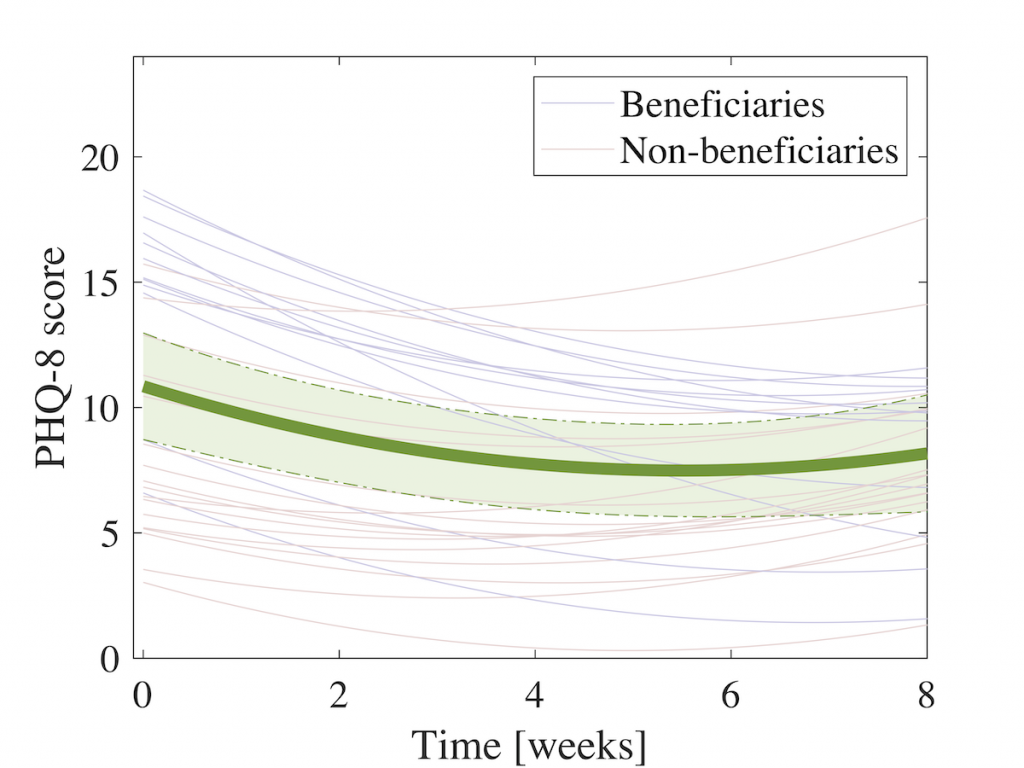
When making a sub-group analysis, we observed that patients who had moderate-to-severe symptoms on study onset had the most benefit of using MUBS over the study period. This is shown in Figure 5.
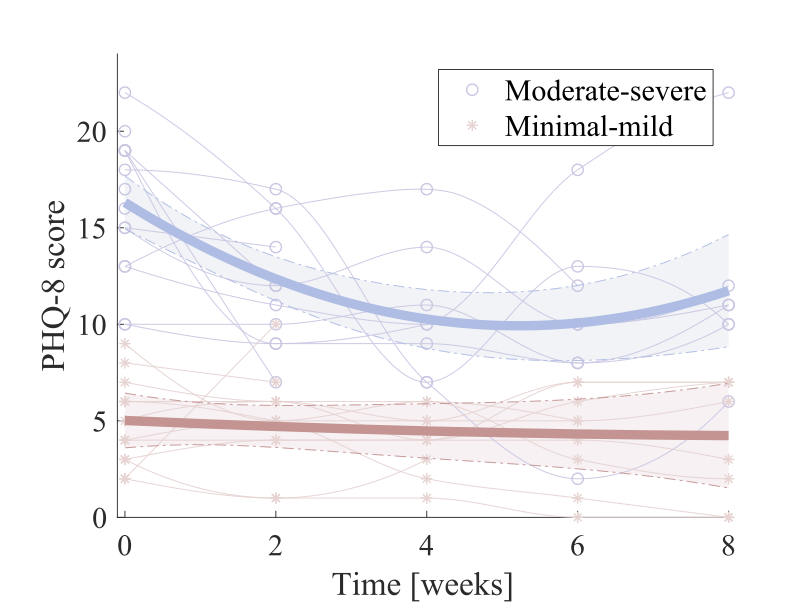
We also did another sub-group analysis differentiating patients who experienced improved depressive symptoms (what we call “beneficiaries“) from those who displayed minimal or no improvement (called “non-beneficiaries“). This analysis helped understand differences in system usage between the two groups. There were two main differences between the two groups.
- First, we saw a more substantial degree of personalization in the beneficiaries. The beneficiaries reduced their overall use of activities from the standard catalog and instead created and scheduled their own individual activities. These activities were mainly within the “daily living” category, such as medication reminders, sleep hygiene activities, or healthy eating.
- Second, the beneficiaries displayed a more diverse activation by scheduling and performing a more balanced and broad set of activities across different types. They took inspiration to plan activities within life areas, which seems to be neglected.
These studies confirms that a smartphone-based approach to BA might be a feasible approach for supporting mental health problems, especially depression. Based on our detailed analysis of what seems to benefit the beneficiaries, we recommend that smartphone-based systems for BA incorporate support for personalization, allowing patients to customize their activation while still having access to the catalog of standardized and generic activities for inspiration. As such, recommender technology may play a central role in helping the patient create a more diversified schedule by creating awareness of different activities and supporting the planning of activities within less explored areas.
#2 – Data Analysis and Mood Predictions
The second part of the RADMIS project has been focusing on using advanced machine learning (ML) techniques for analysing and forecasting of mood. The main method developed for mood prediction is a hierarchical Bayesian regression model, which is a multi-task learning method that is able to account for individual differences [9, 10].
In one study [9], we applied these hierarchical Bayesian regression models to forecast patients’ self-reported mood for up to seven days. This study was based on 15,975 smartphone self-assessments from 84 patients with bipolar disorder. We did two time-series cross-validation 1-day forecast experiments corresponding to two different real-world scenarios and compared the outcomes with commonly used baseline methods. As shown in Figure 5, the proposed hierarchical Bayesian regression model outperformed pooled and separate models in a 7-day forecast time-series cross-validation experiment and achieved the predicted metrics. When increasing the forecast horizon, forecast errors also increased and the forecast regressed toward the mean of data distribution. We also found that the best performing model used a history of 4 days of self-assessment to predict future mood scores with historical mood scores being the most important predictor variable. In other words, the best predictor for a patient’s mood is the patient’s own mood self-assessment for the last 4 days.
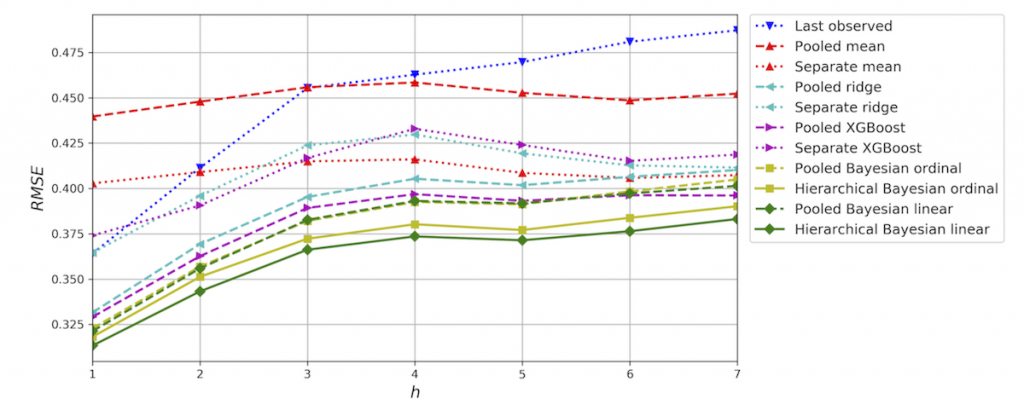
In a second study [10], the proposed ML method was applied to predict clinical ratings of mood, i.e. rating normally done by trained clinicians. In contrast to the above study, forecasting of clinical ratings may be used in clinical diagnosis, which rely on clinical rating scales such as the Hamilton Depression Rating Scale (HDRS) and the Young Mania Rating Scale (YMRS). Frequent automatic estimation of symptom severity based on continuous collection of smartphone data could potentially help support monitoring of illness activity and allow for early treatment intervention between outpatient visits.
Based on a total of 280 clinical ratings collected from 84 patients with bipolar disorder(BD) along with daily smartphone-based self-assessments, we applied the hierarchical Bayesian modelling approach.
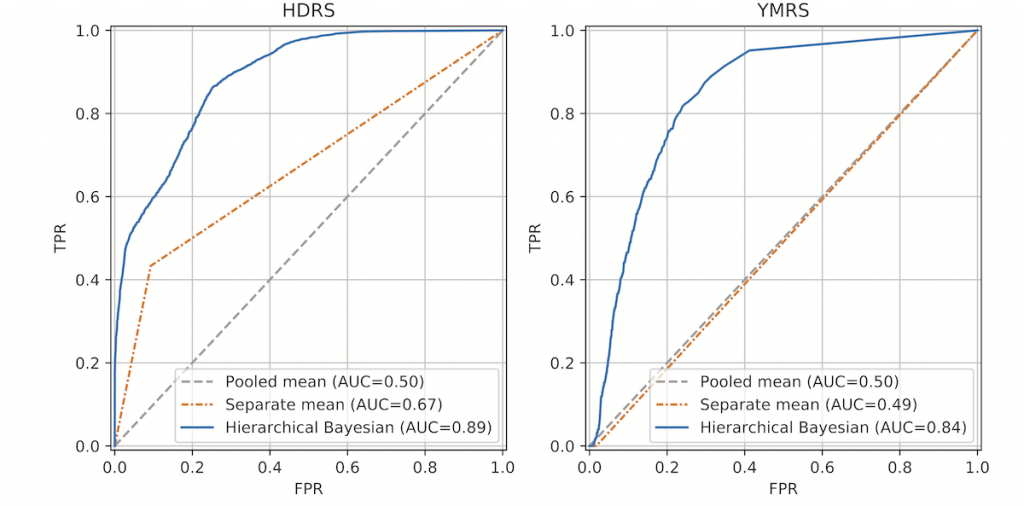
Figure 6 shows receiver operating characteristic (ROC) curves of the HDRS total and the YMRS total models illustrating the trade-off between true positive rate (TPR) and false positive rate (FPR), comparing the hierarchical Bayesian regression model to the naïve pooled and separate mean models. The results show that the hierarchical Bayesian regression model was able to account for information in the smartphone-based self- assessments as well as individual differences and achieved the highest AUC of 0.89 in the HDRS case and 0.84 in the YMRS case. In both cases, smartphone-based self-reported mood was the most important predictor variable – just as we found in the first study described above.
This study shows that daily smartphone-based self-assessments can be utilized to automatically estimate clinical ratings of severity of depression and mania in patients with BD and assist in identifying individuals with high risk of relapse.
#3 – Clinical Evidence and Trial
For over a decade now, there has been a lot of interest in using smartphone technology within mental health, and quite a lot of technologies and systems have been designed and tested [1]. However, limited clinical evidence for the efficacy of these technologies yet exists.
As part of the RADMIS project, we did a systematic literature review of smartphone-based technology for monitoring and treating a range of psychiatric disorders and symptoms [11]. Within medicine, randomized controlled trials (RCTs) are the standard method for providing the evidence of effects. Therefore, the literature review aimed to identify and describe RCTs investigating the effect of smartphone-based treatment in adult patients with a psychiatric diagnosis.
Another recent literature review (2017) included 18 RCT studies regarding depressive symptoms and conducted a meta-analysis on the possible effects of smartphone-based treatment on these symptoms. This study found that taken together across all studies, depressive symptoms were reduced significantly more from smartphone apps than control conditions. However, often, a psychiatric diagnosis was not present – the focus was on depressive symptoms, which were often self-reported.
In our review study, a total of 27 trials comprising 3312 patients within a range of psychiatric diagnoses were included. Among them 10 trials were concerning affective disorders (unipolar and bipolar disorders). The study showed that only 3 of these used a psychiatric diagnosis as outcome measure, whereas the rest relied on self-rating questionnaires. Of these 3 studies, only one study showed significant reductions in depressive symptoms at 6 and 12 weeks. However, these effects were not maintained at 24-weeks follow up.
Hence, very little clinical evidence exists for the efficacy of using smartphone technology for depressive illness. Moreover, the review study also found that the 27 trial designs and reporting were of low quality compared with more classic medical RCTs, and that heterogeneity and methodological issues in individual trials limit the currently available evidence.
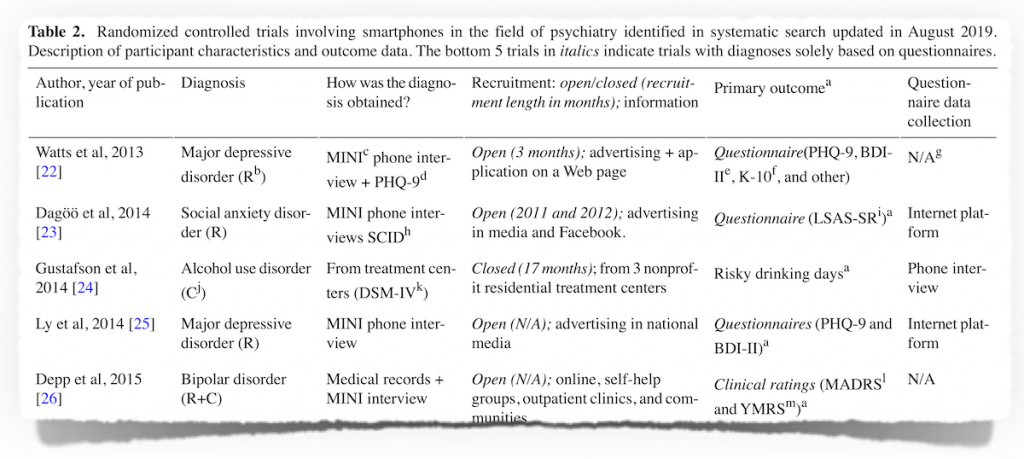
In order to establish clinical evidence for the efficacy for the use of smartphone technology in psychiatry, we initiated the RADMIS Trial [12].
As outlined above, unipolar and bipolar disorder combined account for the highest health care costs of all psychiatric and neurological disorders. Among these, costs due to psychiatric hospitalization is the major part. Several studies – including our own – have shown that smartphones comprise an innovative and unique platform for the monitoring and treatment of depression and mania. However, no prior trial has investigated whether the use of a smartphone-based system can prevent re-admission among patients discharged from hospital. The RADMIS Trial aims to investigate whether using a smartphone-based monitoring and treatment system – including an integrated clinical feedback loop – can reduce the rate and duration of re-admissions compared to standard treatment in unipolar disorder and bipolar disorder.
It should be noted, that in this trial the “smartphone technology” used is the MONARCA-based technology, and not the novel systems (MORIBUS / MUBS) described above. At the time the clinical trial started, these new systems were still in development. The RADMIS clinical trial protocol [12] outlines the background, methods, and outcome measures for the RADMIS clinical study.
We conducted a pragmatic, investigator-blinded, randomized controlled trial. The intervention group received a smartphone-based monitoring and treatment system in addition to standard treatment. The system allowed patients to self-monitor symptoms and access psycho-educative information and cognitive modules. The patients were allocated a study-nurse who, based on the monitoring data, guided and supported them. The control group received standard treatment. The trial lasted six months, with outcome assessments at 0, 3, and 6 months. The outcome measures are:
- Number and duration of re-admissions (primary)
- Severity of depressive and manic symptoms; psychosocial functioning; number of affective episodes (secondary)
- Perceived stress, quality of life, self-rated depressive symptoms, self-rated manic symptoms, recovery, empowerment, adherence to medication, wellbeing, rumination, worrying, and satisfaction (tertiary).
The trial included 120 patients with unipolar depressive disorder (ICD-10) [13]. The results were, unfortunately, rather disappointing since we found no effects on the primary and secondary outcome measures.
As for the primary outcome, intention-to-treat analyses showed no statistically significant differences in time to readmission (Log-Rank p=0.9) or duration of readmissions (B=-16.41,95% CI:-47.32;25.5, p=0.3). As for the secondary outcomes, there were no differences in clinically rated depressive symptoms (p=0.6) or functioning (p=0.1).
However, as for the tertiary outcomes, the intervention group had higher levels of recovery (B=7,29, 95% CI:0.82;13,75, p=0.028) and a tendency towards higher quality of life (p=0.07), wellbeing (p=0.09) satisfaction with treatment (p=0.05) and behavioral activation (p=0.08) compared with the control group.
So in conclusion, we found no effect of the intervention on primary or secondary outcomes. In tertiary outcomes, patients in the intervention group reported higher levels of recovery compared to the control group. All the details is reported in the paper [13].
Looking Ahead
The RADMIS project has resulted in some interesting and promising technological results. Especially the design and deployment of MUBS has shown really promising, with some early result showing reduction in depressive symptoms. Even though our own trials has ended, MUBS is still in active use by quite a number of users, since it is available for both iOS and Android. We are continuing the work on MUBS and will soon release a version 2 of it. This version will still use the CARP Mobile Sensing Framework [14] which has now been significantly improved and updated. The data is now stored and handled in the CACHET Research Platform (CARP). The new version of MUBS will also include the ML-based recommender technology on the phone for even better performance.
The RADMIS clinical trial described above was done on the “old” MONARCA-based smartphone software, and not MUBS. Therefore, the goal is to conduct a new clinical RCT, which will investigate the clinical efficacy of MUBS.
For this continued research on MUBS, we hope to be able to attract further funding for this line of research. We are looking forward to keep you posted on this – in a separate blog post.
Funding
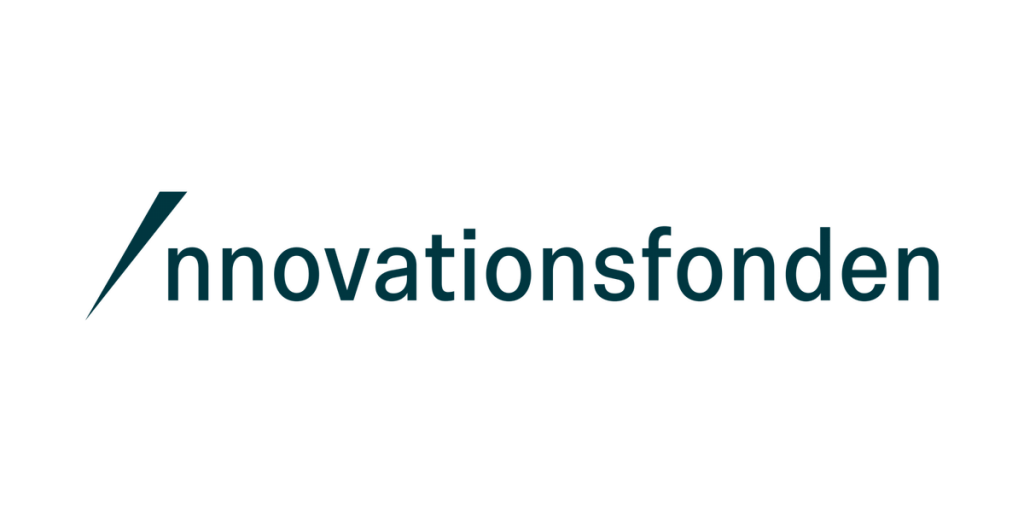
The RADMIS project was funded by the Innovation Fund Denmark.
Partners
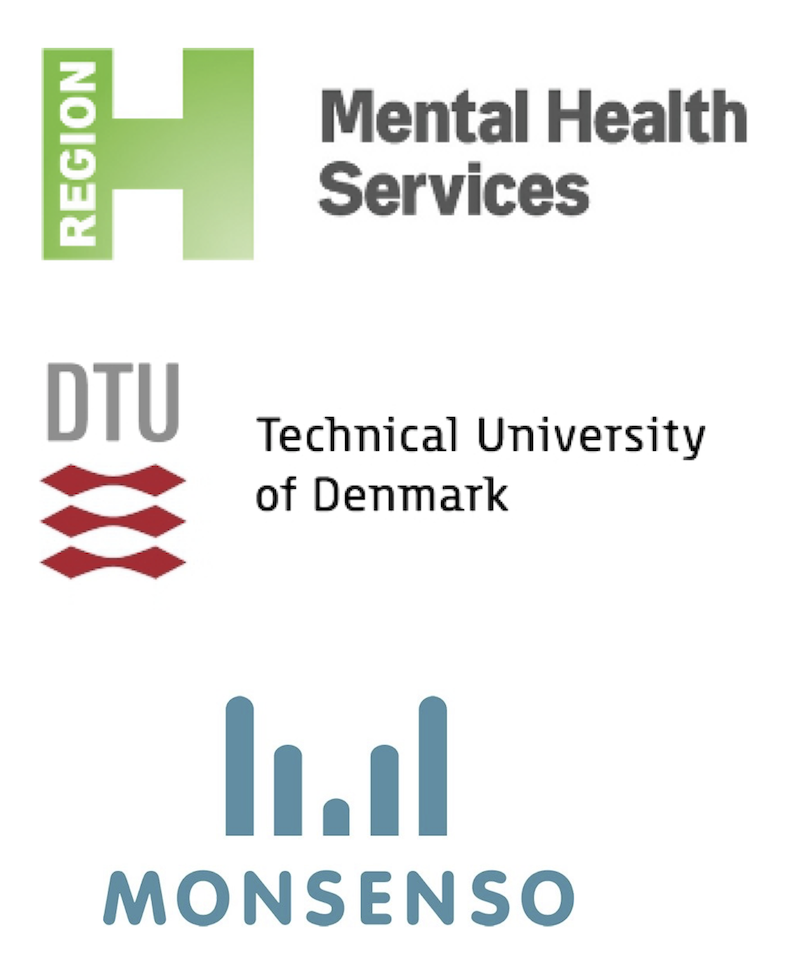
Psychiatric Center Copenhagen, Mental health services in the Capital Region of Denmark (coordinator)
Department of Applied Mathematics and Computer Science, Technical University of Denmark
Department of Health Technology, Technical University of Denmark.
References
![[pdf]](https://www.bardram.net/wp-content/plugins/papercite/img/pdf.png)
![[doi]](https://www.bardram.net/wp-content/plugins/papercite/img/external.png)
[Bibtex]
@article{ieee-pvc-bardram2020,
author = {Bardram, Jakob E and Matic, Alexandar},
doi = {10.1109/MPRV.2019.2925338},
issn = {1558-2590},
journal = {IEEE Pervasive Computing},
pages = {1--11},
title = {{A Decade of Ubiquitous Computing Research in Mental Health}},
year = {2020}
}
![[pdf]](https://www.bardram.net/wp-content/plugins/papercite/img/pdf.png)
![[doi]](https://www.bardram.net/wp-content/plugins/papercite/img/external.png)
[Bibtex]
@article{rohani2018correlations,
author = {Rohani, A Darius and Faurholt-Jepsen, Maria and Kessing, Vedel Lars and Bardram, E Jakob},
doi = {10.2196/mhealth.9691},
file = {:Users/bardram/Documents/Mendeley Desktop/fd000374d85f1e70f1c78103e4d84e5a.pdf:pdf},
issn = {2291-5222},
journal = {JMIR Mhealth Uhealth},
keywords = {affective disorder,behavior,bipolar disorder,correlation,depression,depressive mood symptoms,mobile phone,mood disorder,objective features,sensor data,systematic review,wearable devices},
month = {aug},
number = {8},
pages = {e165},
title = {{Correlations Between Objective Behavioral Features Collected From Mobile and Wearable Devices and Depressive Mood Symptoms in Patients With Affective Disorders: Systematic Review}},
url = {http://mhealth.jmir.org/2018/8/e165/},
volume = {6},
year = {2018}
}
![[pdf]](https://www.bardram.net/wp-content/plugins/papercite/img/pdf.png)
[Bibtex]
@inproceedings{bardram2017supporting,
author = {Bardram, Jakob E and Rohani, Darius A and Tuxen, Nanna and Faurholt-Jepsen, Maria and Kessing, Lars V},
booktitle = {Proceedings of the 2017 ACM International Joint Conference on Pervasive and Ubiquitous Computing and Proceedings of the 2017 ACM International Symposium on Wearable Computers},
file = {:Users/bardram/Documents/Mendeley Desktop/Bardram et al. - 2017 - Supporting smartphone-based behavioral activation a simulation study.pdf:pdf},
keywords = {CBT,Moribus,RADMIS},
mendeley-groups = {RADMIS},
mendeley-tags = {CBT,Moribus,RADMIS},
organization = {ACM},
pages = {830--843},
title = {{Supporting smartphone-based behavioral activation: a simulation study}},
year = {2017}
}
![[pdf]](https://www.bardram.net/wp-content/plugins/papercite/img/pdf.png)
![[doi]](https://www.bardram.net/wp-content/plugins/papercite/img/external.png)
[Bibtex]
@article{rohani2018learning,
abstract = {Background: Behavioral activation is a pen and paper-based therapy form for treating depression. The patient registers their activity hourly, and together with the therapist, they agree on a plan to change behavior. However, with the limited clinical personnel, and a growing patient population, new methods are needed to advance behavioral activation. Objective: The objectives of this paper were to (1) automatically identify behavioral patterns through statistical analysis of the paper-based activity diaries, and (2) determine whether it is feasible to move the behavioral activation therapy format to a digital solution. Methods: We collected activity diaries from seven patients with bipolar depression, covering in total 2,480 hours of self-reported activities. A pleasure score, on a 1-10 rating scale, was reported for each activity. The activities were digitalized into 6 activity categories, and statistical analyses were conducted. Results: Across all patients, movement-related activities were associated with the highest pleasure score followed by social activities. On an individual level, through a nonparametric Wilcoxon Signed-Rank test, one patient had a statistically significant larger amount of spare time activities when feeling bad (z=?2.045, P=.041). Through a within-subject analysis of covariance, the patients were found to have a better day than the previous, if that previous day followed their diurnal rhythm ($\rho$=.265, P=.029). Furthermore, a second-order trend indicated that two hours of daily social activity was optimal for the patients ($\beta$2=?0.08, t (63)=?1.22, P=.23). Conclusions: The data-driven statistical approach was able to find patterns within the behavioral traits that could assist the therapist in as well as help design future technologies for behavioral activation. [JMIR Ment Health 2018;5(2):e10122]},
author = {Rohani, Darius Adam and Tuxen, Nanna and Lopategui, Andrea Quemada and Kessing, Lars Vedel and Bardram, Jakob Eyvind},
doi = {10.2196/10122},
file = {:Users/bardram/Documents/Mendeley Desktop/6d8b61566c0022ef3e27a412f5ffa3c5.pdf:pdf},
issn = {2368-7959},
journal = {JMIR Mental Health},
keywords = {activities,behavior,behavioral activation,bipolar disorder,circadian rhythm,depression,hourly planning,psychotherapy,statistics},
month = {jun},
number = {2},
pages = {e10122},
publisher = {JMIR Mental Health},
title = {{Data-Driven Learning in High-Resolution Activity Sampling From Patients With Bipolar Depression: Mixed-Methods Study}},
url = {http://mental.jmir.org/2018/2/e10122/},
volume = {5},
year = {2018}
}
![[pdf]](https://www.bardram.net/wp-content/plugins/papercite/img/pdf.png)
[Bibtex]
@inproceedings{rohani2019personalizing,
title={Personalizing mental health: A feasibility study of a mobile behavioral activation tool for depressed patients},
author={Rohani, Darius A and Tuxen, Nanna and Lopategui, Andrea Quemada and Faurholt-Jepsen, Maria and Kessing, Lars V and Bardram, Jakob E},
booktitle={Proceedings of the 13th EAI International Conference on Pervasive Computing Technologies for Healthcare},
pages={282--291},
year={2019}
}
![[pdf]](https://www.bardram.net/wp-content/plugins/papercite/img/pdf.png)
[Bibtex]
@inproceedings{rohani2020mubs,
author = {Rohani, Darius A and Lopategui, Andrea Quemada and Tuxen, Nanna and Faurholt-Jepsen, Maria and Kessing, Lars V and Bardram, Jakob E},
booktitle = {Proceedings of the 2020 CHI Conference on Human Factors in Computing Systems},
file = {:Users/bardram/Documents/Mendeley Desktop/3313831.3376879.pdf:pdf},
pages = {1--13},
title = {{MUBS: A Personalized Recommender System for Behavioral Activation in Mental Health}},
year = {2020}
}
![[pdf]](https://www.bardram.net/wp-content/plugins/papercite/img/pdf.png)
[Bibtex]
@article{bardram2020evaluating,
author={Jakob Eyvind Bardram},
journal={IEEE Pervasive Computing},
title={Evaluating Personalized Pervasive Health Technology - But How?},
year={2020},
volume={19},
number={3},
pages={37-44},
}
![[pdf]](https://www.bardram.net/wp-content/plugins/papercite/img/pdf.png)
![[doi]](https://www.bardram.net/wp-content/plugins/papercite/img/external.png)
[Bibtex]
@article{rohani2021benefits,
title={Benefits of Using Activity Recommender Technology for Self-management of Depressive Symptoms},
author={Rohani, Darius A and Faurholt-Jepsen, Maria and Kessing, Lars V and Bardram, Jakob E},
journal={ACM Transactions on Computing for Healthcare},
doi = {https://doi.org/10.1145/3462212},
volume={2},
number={4},
pages={1--21},
year={2021},
publisher={ACM New York, NY, USA}
}
![[pdf]](https://www.bardram.net/wp-content/plugins/papercite/img/pdf.png)
[Bibtex]
@article{busk2020forecasting,
title={Forecasting Mood in Bipolar Disorder From Smartphone Self-assessments: Hierarchical Bayesian Approach},
author={Busk, Jonas and Faurholt-Jepsen, Maria and Frost, Mads and Bardram, Jakob E and Kessing, Lars Vedel and Winther, Ole},
journal={JMIR mHealth and uHealth},
volume={8},
number={4},
pages={e15028},
year={2020},
publisher={JMIR Publications Inc., Toronto, Canada}
}
![[pdf]](https://www.bardram.net/wp-content/plugins/papercite/img/pdf.png)
![[doi]](https://www.bardram.net/wp-content/plugins/papercite/img/external.png)
[Bibtex]
@article{Busk2020,
abstract = {Currently, the golden standard for assessing the severity of depressive and manic symptoms in patients with bipolar disorder (BD) is clinical evaluations using validated rating scales such as the Hamilton Depression Rating Scale 17-items (HDRS) and the Young Mania Rating Scale (YMRS). Frequent automatic estimation of symptom severity could potentially help support monitoring of illness activity and allow for early treatment intervention between outpatient visits. The present study aimed (1) to assess the feasibility of producing daily estimates of clinical rating scores based on smartphone-based self-assessments of symptoms collected from a group of patients with BD; (2) to demonstrate how these estimates can be utilized to compute individual daily risk of relapse scores. Based on a total of 280 clinical ratings collected from 84 patients with BD along with daily smartphone-based self-assessments, we applied a hierarchical Bayesian modelling approach capable of providing individual estimates while learning characteristics of the patient population. The proposed method was compared to common baseline methods. The model concerning depression severity achieved a mean predicted R2 of 0.57 (SD = 0.10) and RMSE of 3.85 (SD = 0.47) on the HDRS, while the model concerning mania severity achieved a mean predicted R2 of 0.16 (SD = 0.25) and RMSE of 3.68 (SD = 0.54) on the YMRS. In both cases, smartphone-based self-reported mood was the most important predictor variable. The present study shows that daily smartphone-based self-assessments can be utilized to automatically estimate clinical ratings of severity of depression and mania in patients with BD and assist in identifying individuals with high risk of relapse.},
author = {Busk, Jonas and Faurholt-Jepsen, Maria and Frost, Mads and Bardram, Jakob E and Kessing, Lars Vedel and Winther, Ole},
doi = {10.1038/s41398-020-00867-6},
issn = {2158-3188},
journal = {Translational Psychiatry},
number = {1},
pages = {194},
title = {{Daily estimates of clinical severity of symptoms in bipolar disorder from smartphone-based self-assessments}},
url = {https://doi.org/10.1038/s41398-020-00867-6},
volume = {10},
year = {2020}
}
![[pdf]](https://www.bardram.net/wp-content/plugins/papercite/img/pdf.png)
[Bibtex]
@article{tonning2019methodological,
author = {Tonning, Morten Lindbjerg and Kessing, Lars Vedel and Bardram, Jakob Eyvind and Faurholt-Jepsen, Maria},
journal = {Journal of medical Internet research},
number = {10},
pages = {e15362},
publisher = {JMIR Publications Inc., Toronto, Canada},
title = {{Methodological Challenges in Randomized Controlled Trials on Smartphone-Based Treatment in Psychiatry: Systematic Review}},
volume = {21},
year = {2019}
}
![[pdf]](https://www.bardram.net/wp-content/plugins/papercite/img/pdf.png)
![[doi]](https://www.bardram.net/wp-content/plugins/papercite/img/external.png)
[Bibtex]
@article{radmisprotocol2017,
abstract = {Unipolar and bipolar disorder combined account for nearly half of all morbidity and mortality due to mental and substance use disorders, and burden society with the highest health care costs of all psychiatric and neurological disorders. Among these, costs due to psychiatric hospitalization are a major burden. Smartphones comprise an innovative and unique platform for the monitoring and treatment of depression and mania. No prior trial has investigated whether the use of a smartphone-based system can prevent re-admission among patients discharged from hospital. The present RADMIS trials aim to investigate whether using a smartphone-based monitoring and treatment system, including an integrated clinical feedback loop, reduces the rate and duration of re-admissions more than standard treatment in unipolar disorder and bipolar disorder.},
author = {Faurholt-Jepsen, Maria and Frost, Mads and Martiny, Klaus and Tuxen, Nanna and Rosenberg, Nicole and Busk, Jonas and Winther, Ole and Bardram, Jakob Eyvind and Kessing, Lars Vedel},
doi = {10.1186/s13063-017-2015-3},
issn = {1745-6215},
journal = {Trials},
month = {jun},
number = {1},
pages = {277},
title = {{Reducing the rate and duration of Re-ADMISsions among patients with unipolar disorder and bipolar disorder using smartphone-based monitoring and treatment -- the RADMIS trials: study protocol for two randomized controlled trials}},
url = {https://doi.org/10.1186/s13063-017-2015-3},
volume = {18},
year = {2017}
}
![[pdf]](https://www.bardram.net/wp-content/plugins/papercite/img/pdf.png)
![[doi]](https://www.bardram.net/wp-content/plugins/papercite/img/external.png)
[Bibtex]
@article{tonning2021radmis,
author = {Tonning, Morten Lindbjerg and Faurholt-Jepsen, Maria and Frost, Mads and Martiny, Klaus and Tuxen, Nanna and Rosenberg, Nicole and Busk, Jonas and Winther, Ole and Melbye, Sigurd Arne and Thaysen-Petersen, Daniel and Aamund, Kate Andreasson and Tolderlundh, Lizzie and Bardram, Jakob Eyvind and Kessing, Lars Vedel},
doi = {https://doi.org/10.1016/j.jad.2020.12.141},
issn = {0165-0327},
journal = {Journal of Affective Disorders},
keywords = {Depression,Intervention,Randomized controlled trial,Smartphone,Technology,Unipolar depressive disorder},
pages = {354--363},
title = {{The effect of smartphone-based monitoring and treatment on the rate and duration of psychiatric readmission in patients with unipolar depressive disorder: The RADMIS randomized controlled trial}},
url = {http://www.sciencedirect.com/science/article/pii/S0165032720332316},
volume = {282},
year = {2021}
}
![[pdf]](https://www.bardram.net/wp-content/plugins/papercite/img/pdf.png)
[Bibtex]
@article{bardram2020carp,
title={The CARP Mobile Sensing Framework--A Cross-platform, Reactive, Programming Framework and Runtime Environment for Digital Phenotyping},
author={Bardram, Jakob E},
journal={arXiv preprint arXiv:2006.11904},
arxivId = {cs.HC/2006.11904},
year={2020}
}